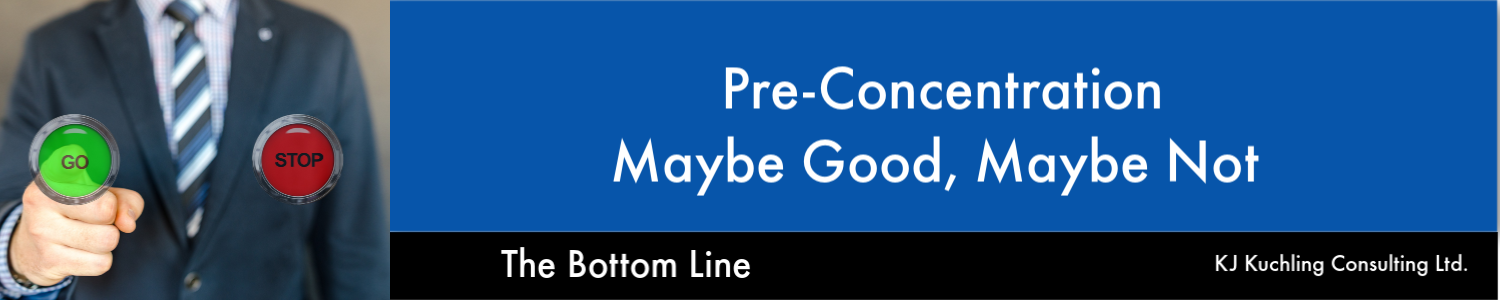
A while back I wrote a blog titled “Pre-Concentration – Savior or Not?”. That blog was touting the benefits of pre-concentration. More recently I attended a webinar where the presenter stated that the economics of pre-concentration may not necessarily be as good as we think they are.
My first thought was “this is blasphemy”. However upon further reflection I wondered if it’s true. To answer that question, I modified one of my old cashflow models from a Zn, Pb project using pre-concentration. I adjusted the model to enable running a trade-off, with and without pre-con by varying cost and recovery parameters.
Main input parameters
The trade-off model and some of the parameters are shown in the graphic below. The numbers used in the example are illustrative only, since I am mainly interested in seeing what factors have the greatest influence on the outcome.
The term “mass pull” is used to define the quantity of material that the pre-con plant pulls and sends to the grinding circuit. Unfortunately some metal may be lost with the pre-con rejects. The main benefit of a pre-con plant is to allow the use of a smaller grinding/flotation circuit by scalping away waste. This will lower the grinding circuit capital cost, albeit slightly increase its unit operating cost.
Concentrate handling systems may not differ much between model options since roughly the same amount of final concentrate is (hopefully) generated.
Another one of the cost differences is tailings handling. The pre-con rejects likely must be trucked to a final disposal location while flotation tails can be pumped. I assumed a low pumping cost, i.e to a nearby pit.
The pre-con plant doesn’t eliminate a tailings pond, but may make it smaller based on the mass pull factor. The most efficient pre-concentration plant from a tailings handling perspective is shown on the right.
The outcome
The findings of the trade-off surprised me a little bit. There is an obvious link between pre-con mass pull and overall metal recovery. A high mass pull will increase metal recovery but also results in more tonnage sent to grinding. At some point a high mass pull will cause one to ask what’s the point of pre-con if you are still sending a high percentage of material to the grinding circuit.
The table below presents the NPV for different mass pull and recovery combinations. The column on the far right represents the NPV for the base case without any pre-con plant. The lower left corner of the table shows the recovery and mass pull combinations where the NPV exceeds the base case. The upper right are the combinations with a reduction in NPV value.
The width of this range surprised me showing that the value generated by pre-con isn’t automatic. The NPV table shown is unique to the input assumptions I used and will be different for every project.
The economic analysis of pre-concentration does not include the possible benefits related to reduced water and energy consumption. These may be important factors for social license and permitting purposes, even if unsupported by the economics. Here’s an article from ThermoFisher on this “How Bulk Ore Sorting Can Reduce Water and Energy Consumption in Mining Operations“.
Conclusion
The objective of this analysis isn’t to demonstrate the NPV of pre-concentration. The objective is to show that pre-concentration might or might not make sense depending on a project’s unique parameters. The following are some suggestions:
1. Every project should at least take a cursory look at pre-concentration to see if it is viable. This should be done on all projects, even if it’s only a cursory mineralogical assessment level.
2. Make certain to verify that all ore types in the deposit are amenable to the same pre-concentration circuit. This means one needs to have a good understanding of the ore types that will be encountered.
3. Anytime one is doing a study using pre-concentration, one should also examine the economics without it. This helps to understand the economic drivers and the risks. You can then decide whether it is worth adding another operating circuit in the process flowsheet that has its own cost and performance risk. The more processing components added to a flow sheet, the more overall plant availability may be effected.
4. The head grade of the deposit also determines how economically risky pre-concentration might be. In higher grade ore bodies, the negative impact of any metal loss in pre-concentration may be offset by accepting higher cost for grinding (see chart on the right).
5. In my opinion, the best time to decide on pre-con would be at the PEA stage. Although the amount of testing data available may be limited, it may be sufficient to assess whether pre-con warrants further study.
6. Don’t fall in love with or over promote pre-concentration until you have run the economics. It can make it harder to retract the concept if the economics aren’t there.